What happens when we run out of data? Or we can't use some valuable datasets due to privacy issues.
What will our AI models learn from once we hit "peak data"? Maybe we'll never reach peak data, same as we never reached "peak oil".
But we're hearing rumbles of AI models running out of internet to train on.
Enter Synthetic Data. The category has emerged as a picks-and-shovels play for those looking to invest in the AI boom without trying to pick an LLM player or pay exorbitant prices.
Well, maybe some exorbitant prices - Scale AI is in talks with Accel to raise hundreds of millions at a $13B valuation (up from $7.3B in 2021) but they're rumored to be doing $675M in Revenue which would give it at ~20x multiple, which in AI land isn't crazy. Scale sells its data generation services to leading AI model companies like OpenAI, Cohere, and Nvidia.
As we'll see below, the synthetic data category has gone from an interesting new tech category in the late 2010s to a critical enabling technology for advanced AI research and ML modeling.
Table of Contents:
- Introduction to Synthetic Data
- End Markets & Use Cases
- Synthetic Data Market Map
- Investment Considerations
"When it comes to GAN models, they are called âadversarialâ networks due to the fact that GANs are actually two networks that compete with each other. The generator is responsible for generating synthetic data, while the second network (the discriminator) operates by comparing the generated data with a real dataset and tries to determine which data is fake. When the discriminator catches fake data, the generator is notified of this and it makes changes to try and get a new batch of data by the discriminator. In turn, the discriminator becomes better and better at detecting fakes. The two networks are trained against each other, with fakes becoming more lifelike all the time."
- In scenarios where real data is limited, biased, or outdated, synthetic data provides a way to generate realistic data for analysis, forecasting, and decision-making. For example, generating synthetic patient data for medical research or financial data for risk modeling.
- Industries dealing with sensitive personal data like healthcare, finance, and telecommunications can leverage synthetic data to adhere to data privacy regulations (GDPR, HIPAA, etc.).
- Synthetic tech firms help their customers by building a synthetic data lake and impose the "shape" of the original data onto a set of synthetic or fake data, as discussed by the Chief Commercial Officer at MDClone in this clip.
â¶ïž Gretel.ai, a synthetic data company based in Palo Alto, which raised a $50M Series B in 2021, shows below how customers can leverage their platform to perform analyses that yield reliable insights leveraging their synthetic data platform.
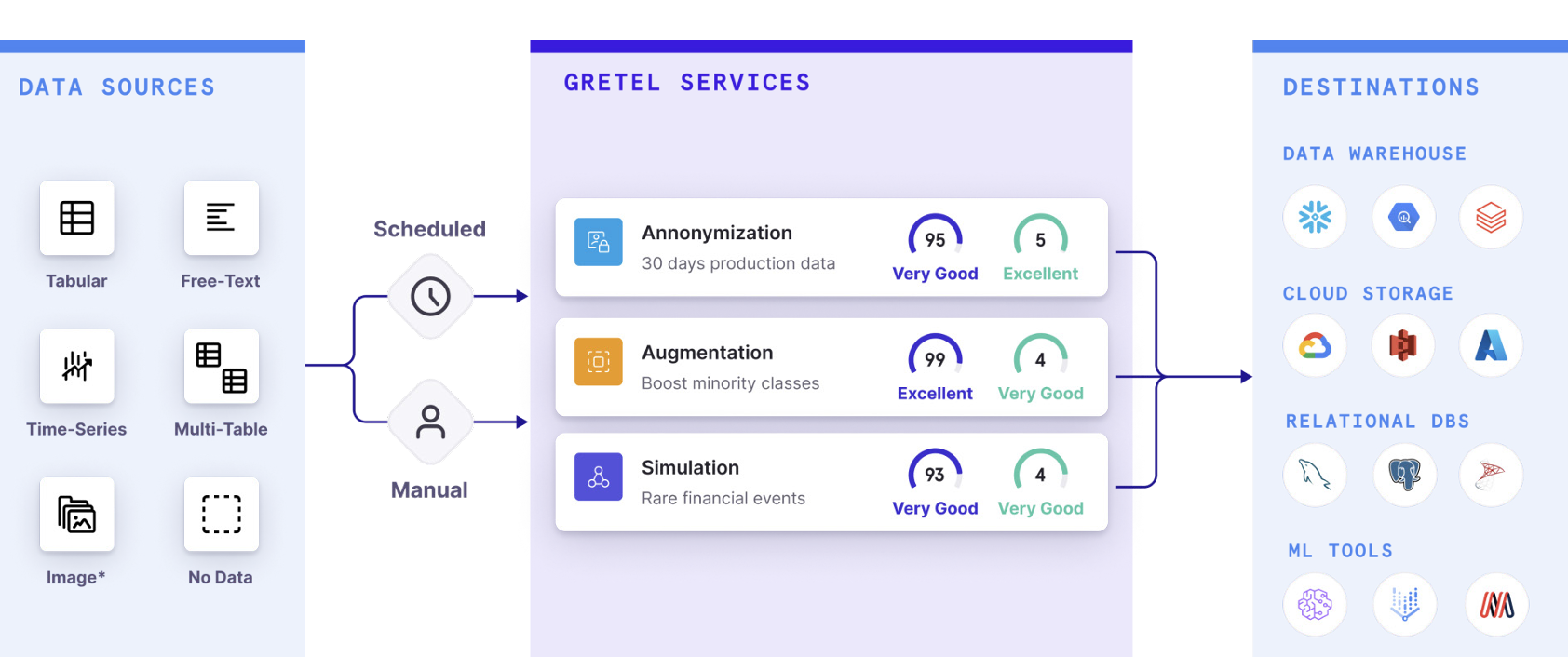
đ° The synthetic data market was already growing before the latest AI boom in 2022-2024 as evidenced by the tangential Digital Twins market having a CAGR of 58% which was projected to reach $50 billion by 2025, according to McKinsey.
The recent frenzy to develop and deploy AI models has rapidly pulled forward demand. According to Gartner, in 2021 only 1% of all data used to train AI was synthetic but they predict that number could be up to 60% in 2024.
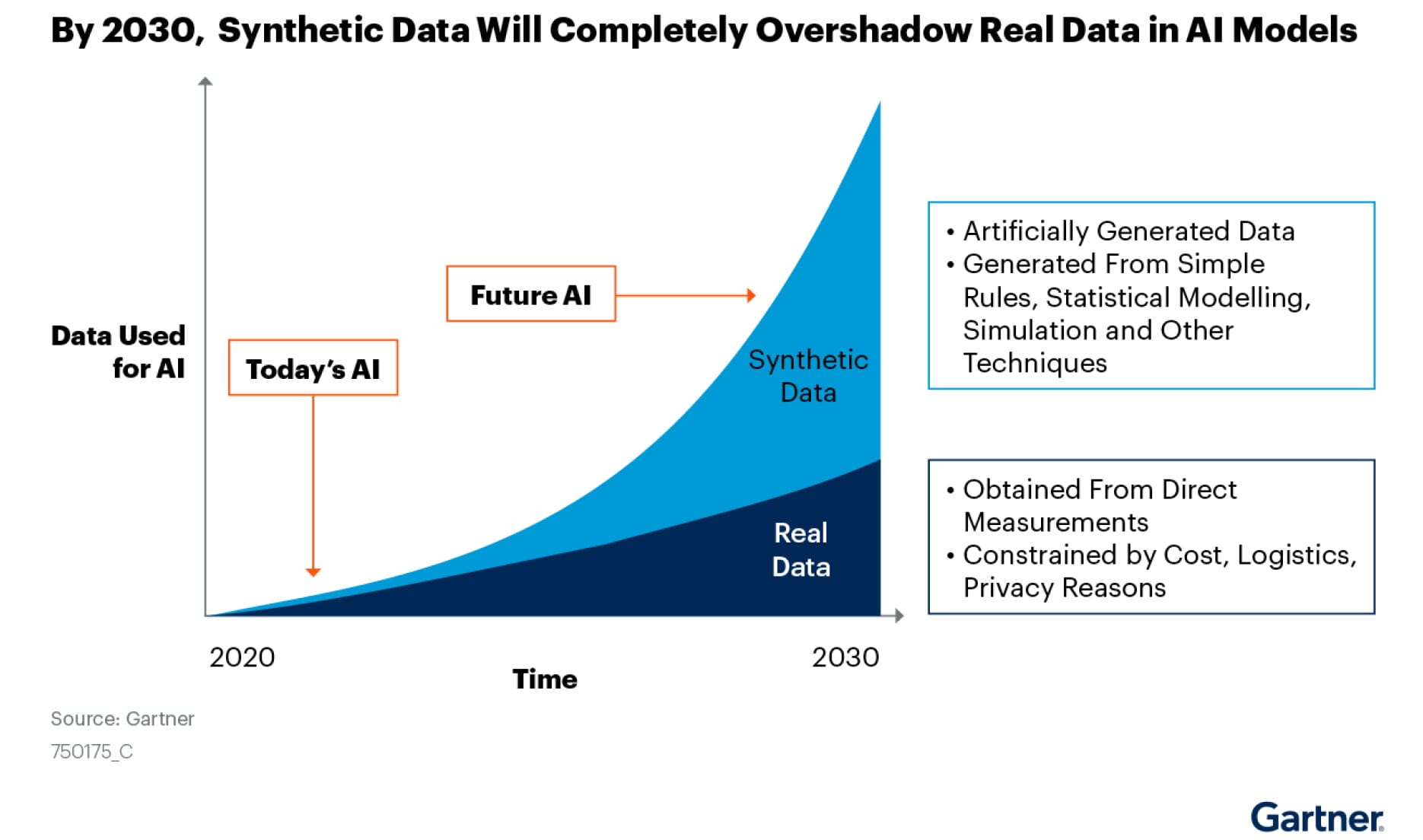
âŹïž In the following sections, we highlight a few companies that are well-positioned to become market leaders and take advantage of AI's voracious demand for data.
End Markets & Use Cases
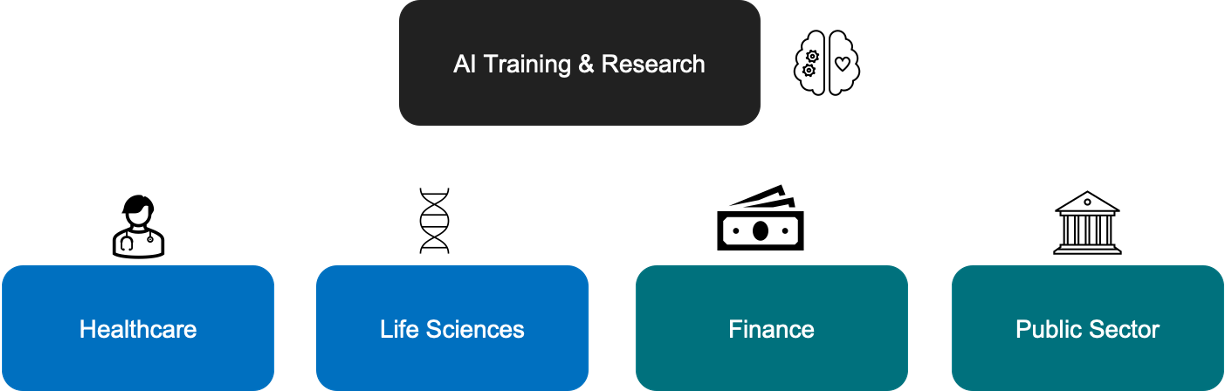
Training AI/ML Models
One of the primary use cases of synthetic data is training AI and machine learning models when real data is scarce, sensitive, or difficult to obtain. Synthetic data can augment or replace real data for tasks like:
- Training computer vision models on synthetic images/videos for object detection, segmentation, etc.
- Training natural language processing models on synthetic text data.
- Synthetic data comes with perfect ground truth annotations, eliminating the need for manual labeling which is time-consuming, expensive and prone to errors.
Healthcare & Life Sciences
Synthetic health data accelerates clinical research while protecting patient privacy by generating artificial records that resemble real EHR data without including any actual patient information.
Organizations also generate synthetic data to augment AI training datasets by boosting low sample sizes, balancing between classes, filling in missing fields, and simulating new examples for underrepresented medical conditions.
- Synthetic healthcare data can help in simulating patient data for clinical trials, facilitating more efficient initial testing phases and protocol development.
- Enable the safe analysis of electronic health records (EHR) by using synthetic patient data, maintaining patient privacy while extracting valuable insights for healthcare improvement.
- Reduce the time required to access data
Some of the largest Healthcare and Insurance providers have begun exploring how to use synthetic data. Anthem stated in 2022 that it was working with Alphabet to create a platform for synthetic data to validate and train AI algorithms that identify things like fraudulent claims or abnormalities in a personâs health records, and those AI algorithms will then be able to run on real-world member data.
The plan is to use algorithms and statistical models to generate approximately 1.5 to 2 petabytes of synthetic data, including artificially generated data sets of medical histories, healthcare claims and other key medical data, created in partnership with Google Cloud.
The ultimate goal, he said, is to validate and train AI algorithms on large amounts of data, while reducing privacy issues surrounding personal medical information - Anil Bhatt, CIO Anthem/Elevance.
Finance & Public Sector
Synthetic data usage offers financial organizations a privacy-preserving solution to enhance risk assessment, fraud detection, algorithm training and software development.
- For Banks: Improve fraud, anti-money-laundring and anomaly detection models
- For Insurers: Personalized customer insights based on high-quality synthetic data
- For FinTechs: Secure algorithm training by maximizing the data and minimizing the privacy risks.
- Regulatory compliance with data protection regulations
- Population Health & Security analyses around image identification for satellite imagery
Below are a few examples of how leading synthetic data companies are servicing their customers.
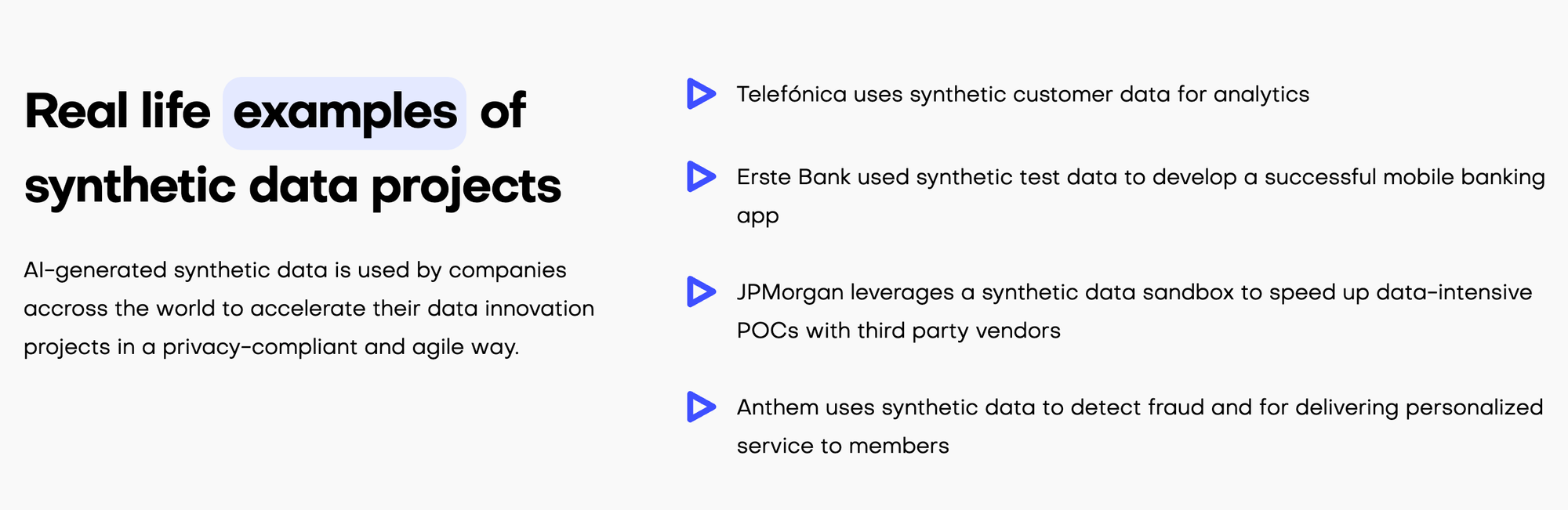
Syntho.ai, a startup based in the Netherlands, has seen considerable market pull from the banking sector across the globe for its synthetic data products, highlighting the global nature of the opportunity for vendors in the space.
Syntheticaic, sells its Rapid Automation Image Categorization (RAIC) platform to customers looking to analyze large sets of geospatial data. Using the RAIC platform they were the first company to find the infamous Chinese spy balloon by analyzing satellite imagery. RAIC found the ballon in two minutes. The company raised a $15M Series B in Feb 2024, with IBM Ventures and Booz Allen participating.
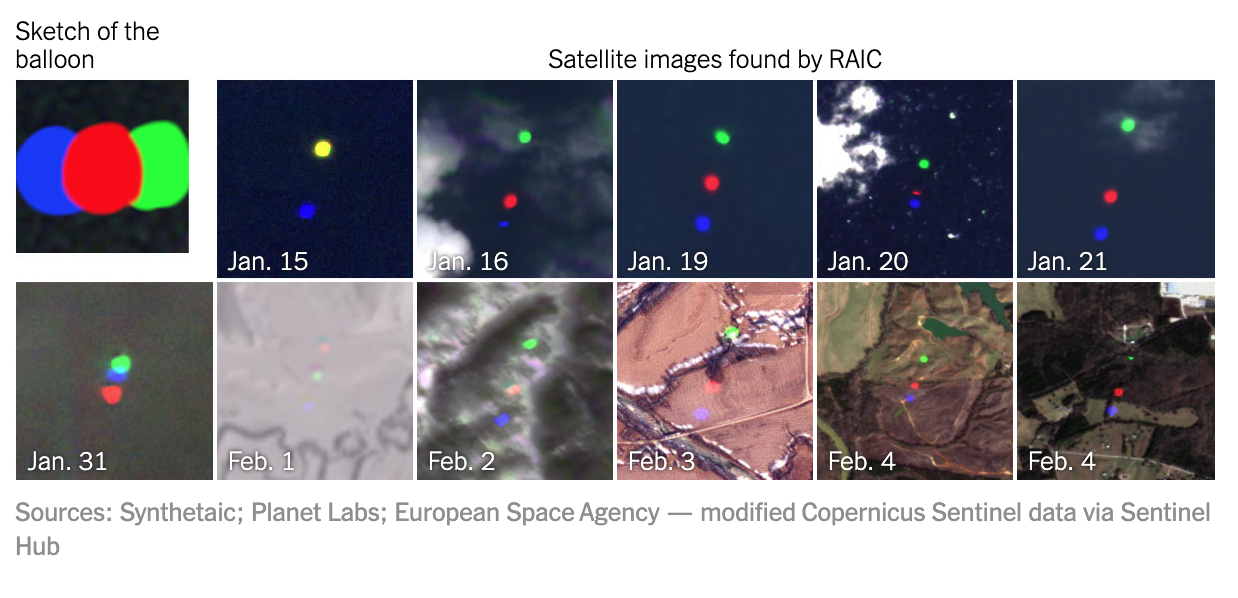
Epistemix, a synthetic data company based in Salt Lake City, built its initial product around public health simulations. As described by their CEO & Co-Founder, John Cordier:
our software is like a hyper-realistic SimCity where you can evaluate the impact strategies, policies, and interventions have on the populations and places in the simulation before you take action in the real world.
The current iteration of their product allows customers to create agent-based models to simulate outcomes of policy decisions and is built on top of a synthetic population of the United States that is statistically accurate down to the census block level.
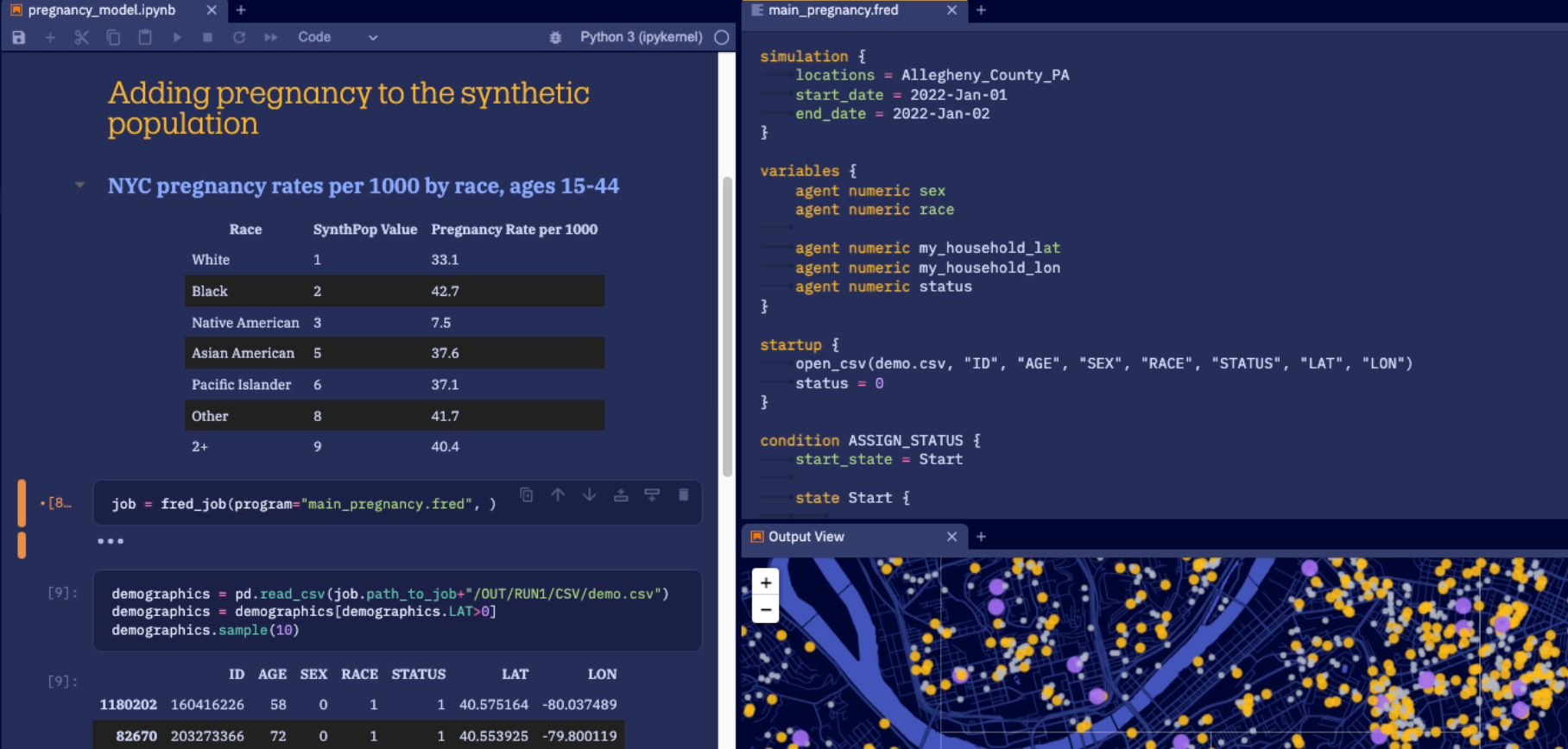
Synthetic Data Market Map
There's been significant activity in the space over the last few years, with the first generation of companies carving out niches in specific end markets (Banking, Healthcare, etc.)
- A lot of companies started in Europe in response to the GDPR rules forcing EU companies and US companies with European consumer data to tread more carefully when analyzing their data (e.g, Mostly AI).
- The next generation of companies in the space are looking to take advantage of the recent explosion in AI companies and models, positioning themselves as more software infrastructure companies (e.g., Tonic.ai)
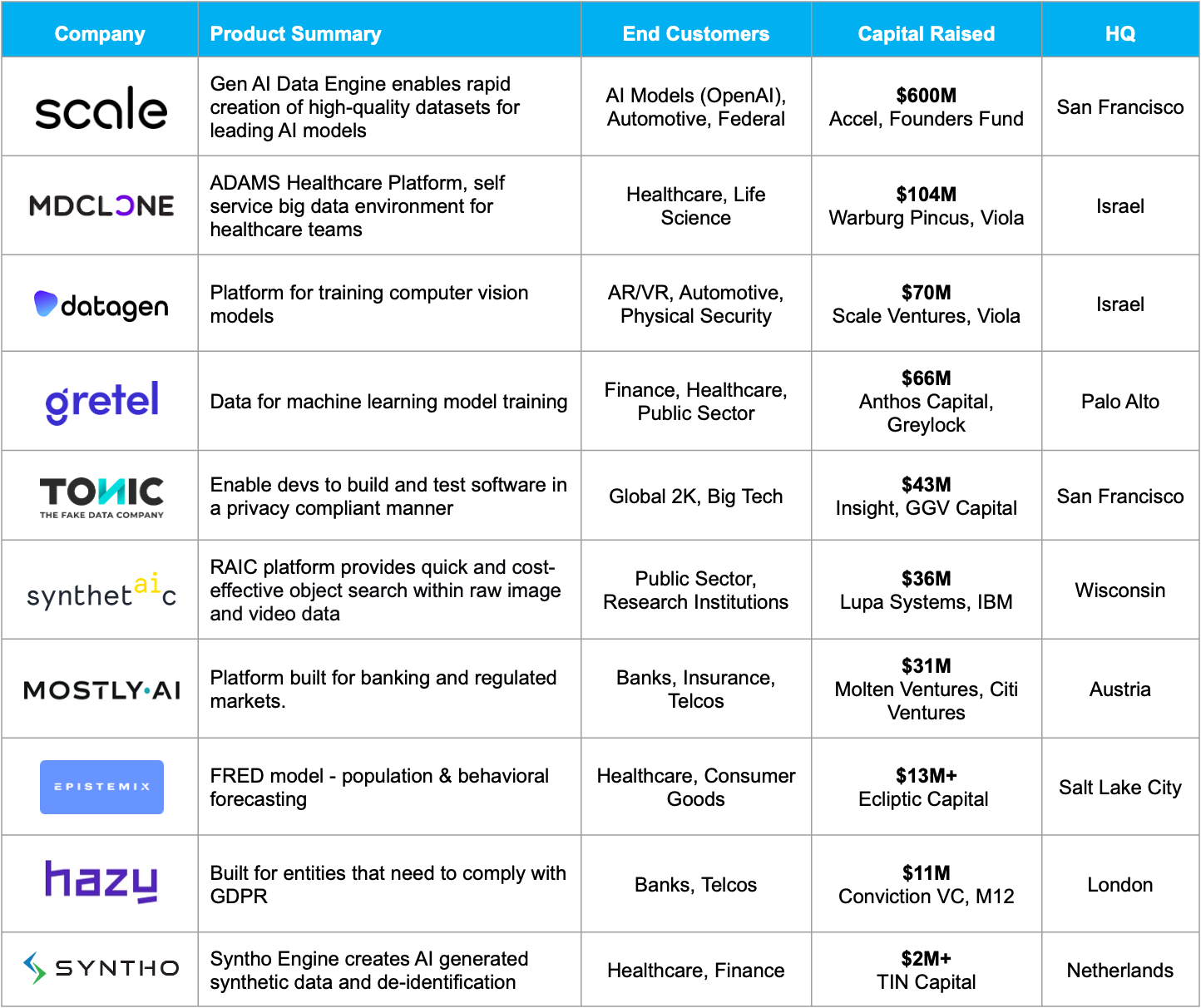
Mostly AI has an active blog commenting on the space, below they've outlined a more exhaustive list of competitors and players entering the space.
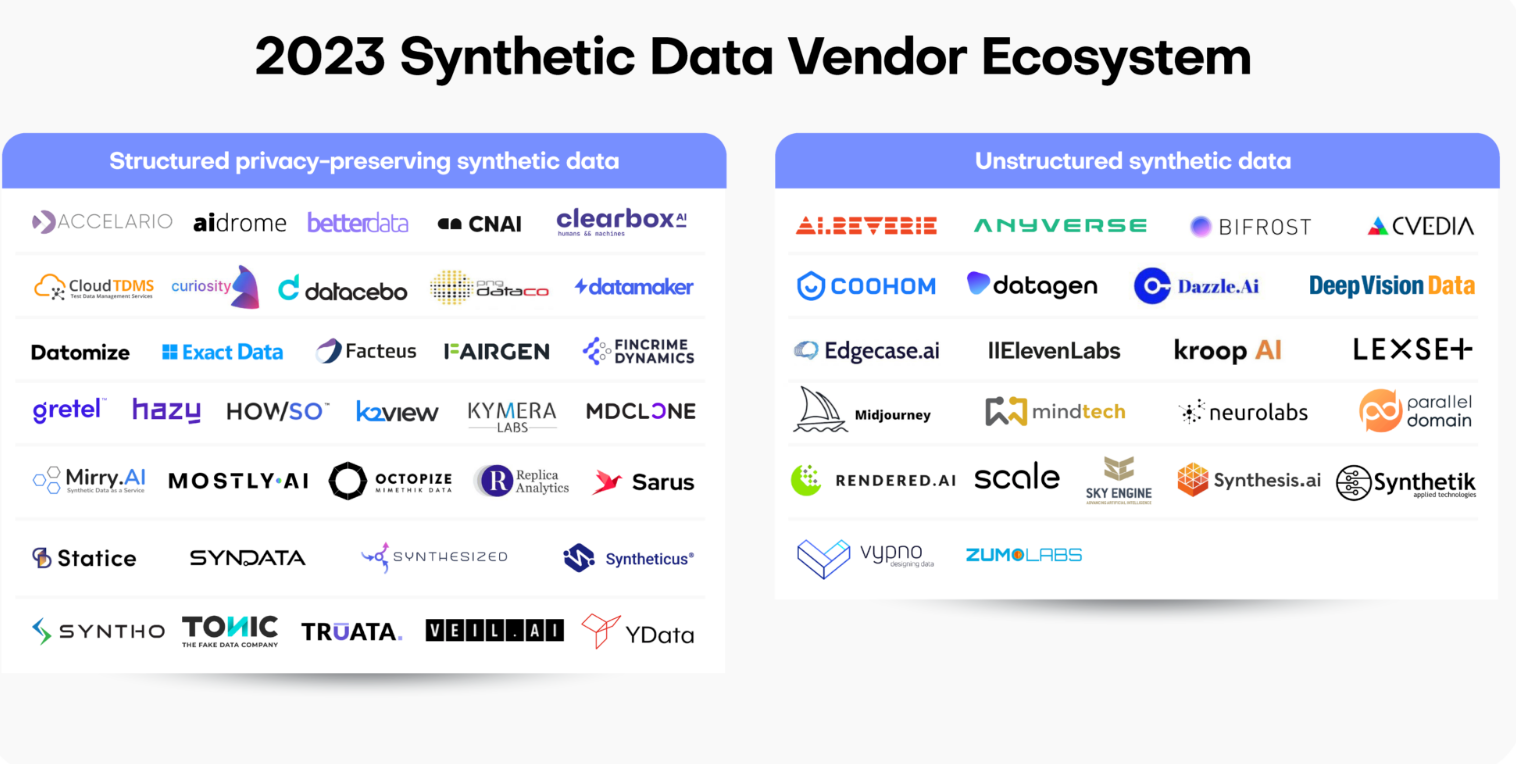
Some of these companies are classified by Matt Turk at FirstMark in his annual The 2024 MAD (ML, AI & Data) Landscape in the 'Data Generation & Labelling' category.
Investment Considerations
Despite what looks like an attractive market, synthetic data isn't without its limitations and there remain key risks and open questions for anyone investing or building in the category.
âQuality of Synthetic Data and ability to draw inferences
- Synthetic data may not capture the complexity of real-world datasets and can potentially omit important details or relationships needed for accurate predictions.
- A 1B parameter synthetic data set based on a 10M parameter real data set may generate insights that are incrementally helpful on those 10M parameters but said insights could be vastly different when compared to a 1B parameter real data set.
đ§ Disintermediation Risk from Companies closer to the data
- Platforms and technologies, such as data warehouses/lakes, that sit closer to customers' data may build their own synthetic data tooling which would disintermediate some of the pure play synthetic data vendors
- Modern Healthcare Data platforms and EHRs such as Zus, and Tuva offer synthetic patient data tools for Health Tech companies to run analyses on their patient population.
- Larger software vendors may scoop up smaller teams via M&A or partnerships, increasing competition and leaving synthetic players at risk of being viewed as a feature (ex: Aetion acquired Replica, Facebook acquired AI.Reverie and Datavant acquired Syntegra)
đ„ Competitive Market with at least 5 companies raising $40M+
- Questionable as to whether we would need net new Seed or Series A stage companies in the space unless there is a unique angle
- Identifying technical differentiation among competitors becoming more difficult
đ” Consumption-based pricing models may make predicting revenue difficult
- Larger vendors will be able to require minimum spends but smaller companies may struggle to generate enough revenue to warrant investment.
- Price erosion possible overtime due to competition and commoditization of technology
- Tech lends itself to requiring services/onboarding which may lower gross margins until fully self-service models are available
If you are investing or building in the space please reach out at michael@laborcapital.co if you'd like to review deal opportunities or provide additional commentary to the article.
Contributing Editor: Thanks to Alan Shi, a sophomore at Washington University in St. Louis, for his help researching this article. Alan is part of the Labor Capital Fellows Program.
Additional Resources


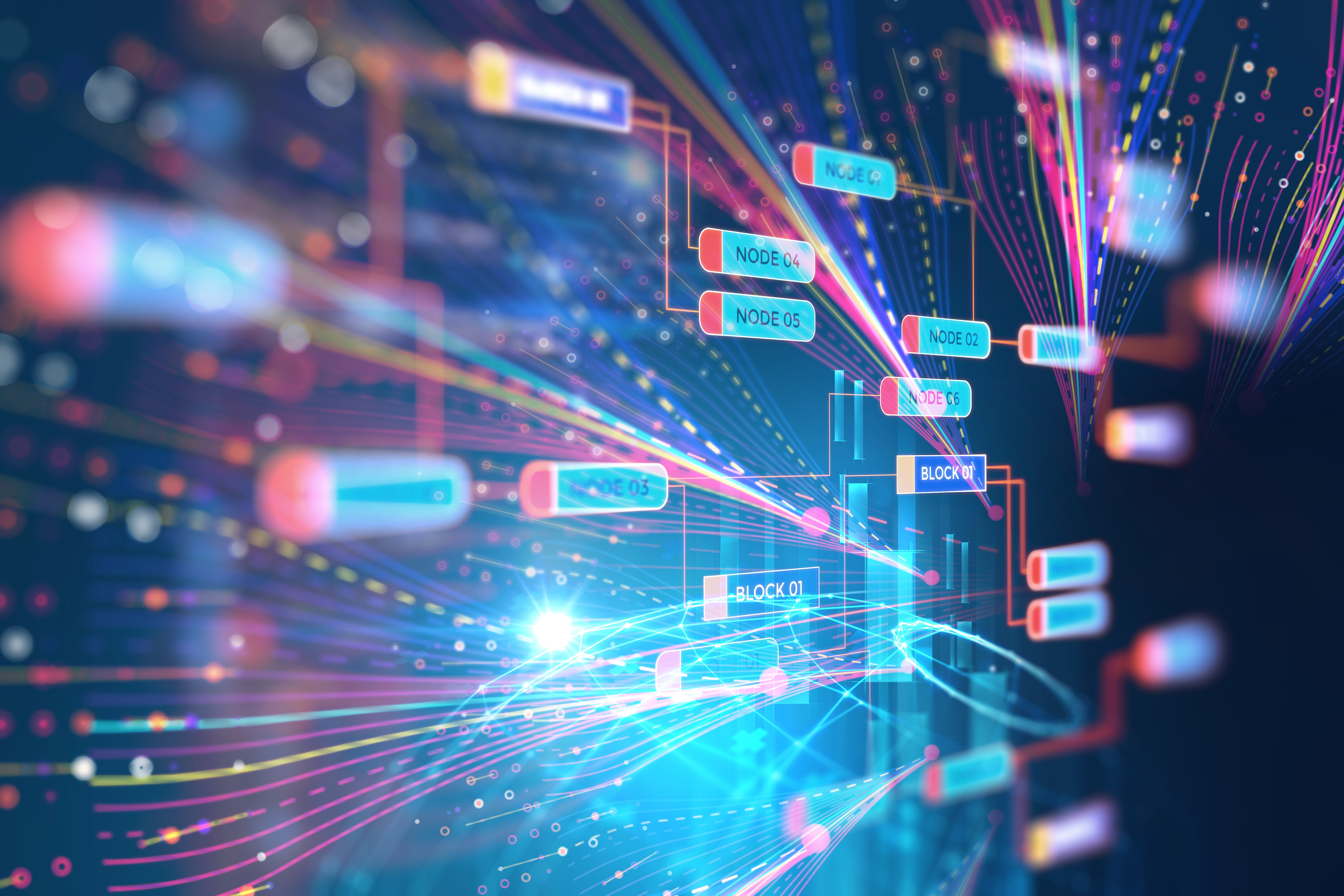


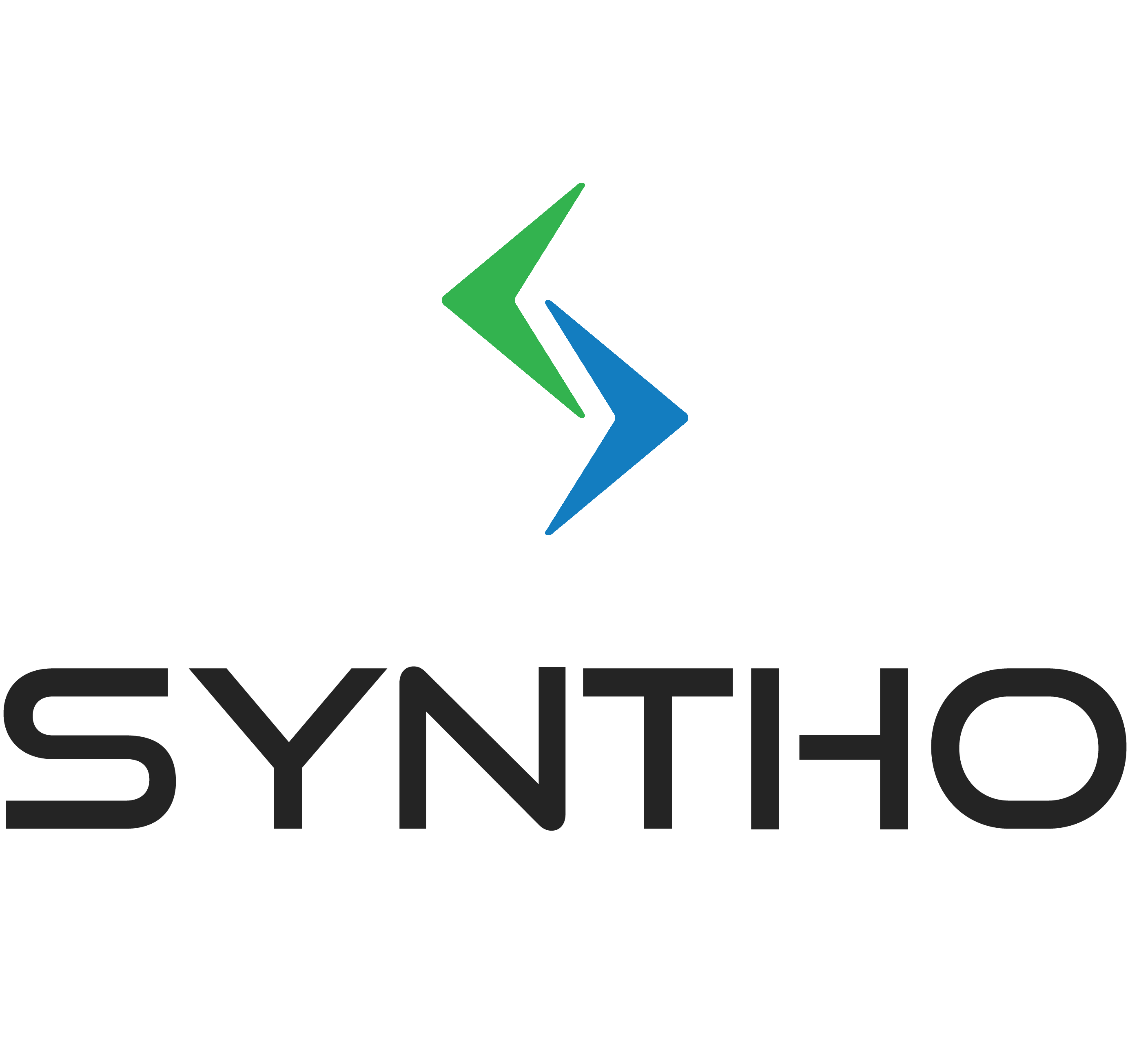
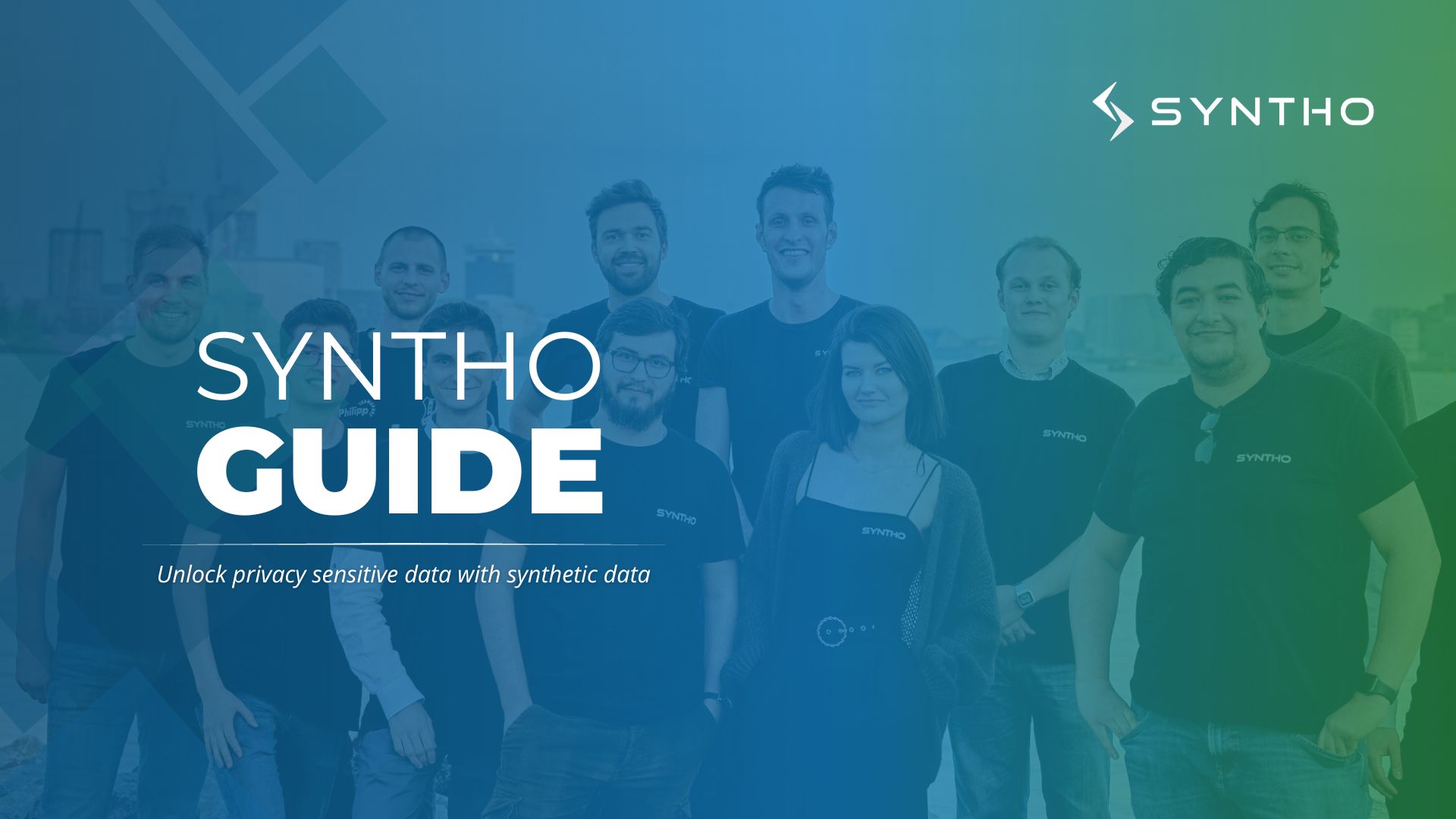
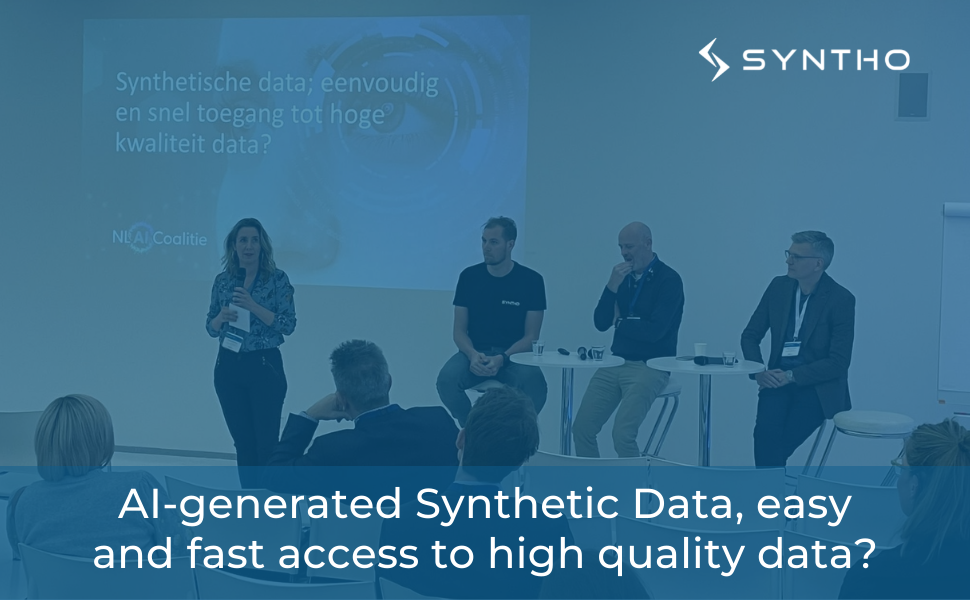
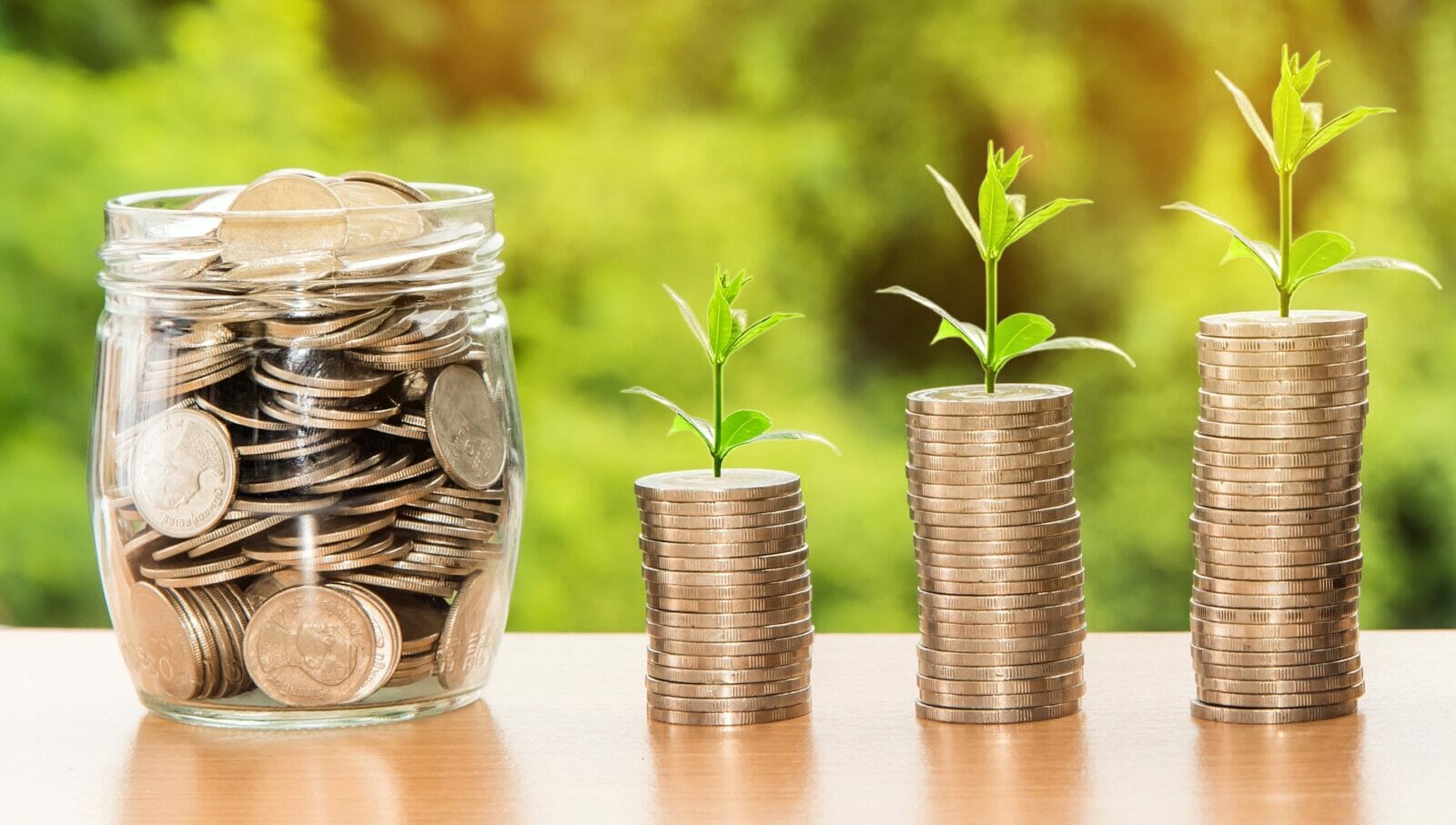
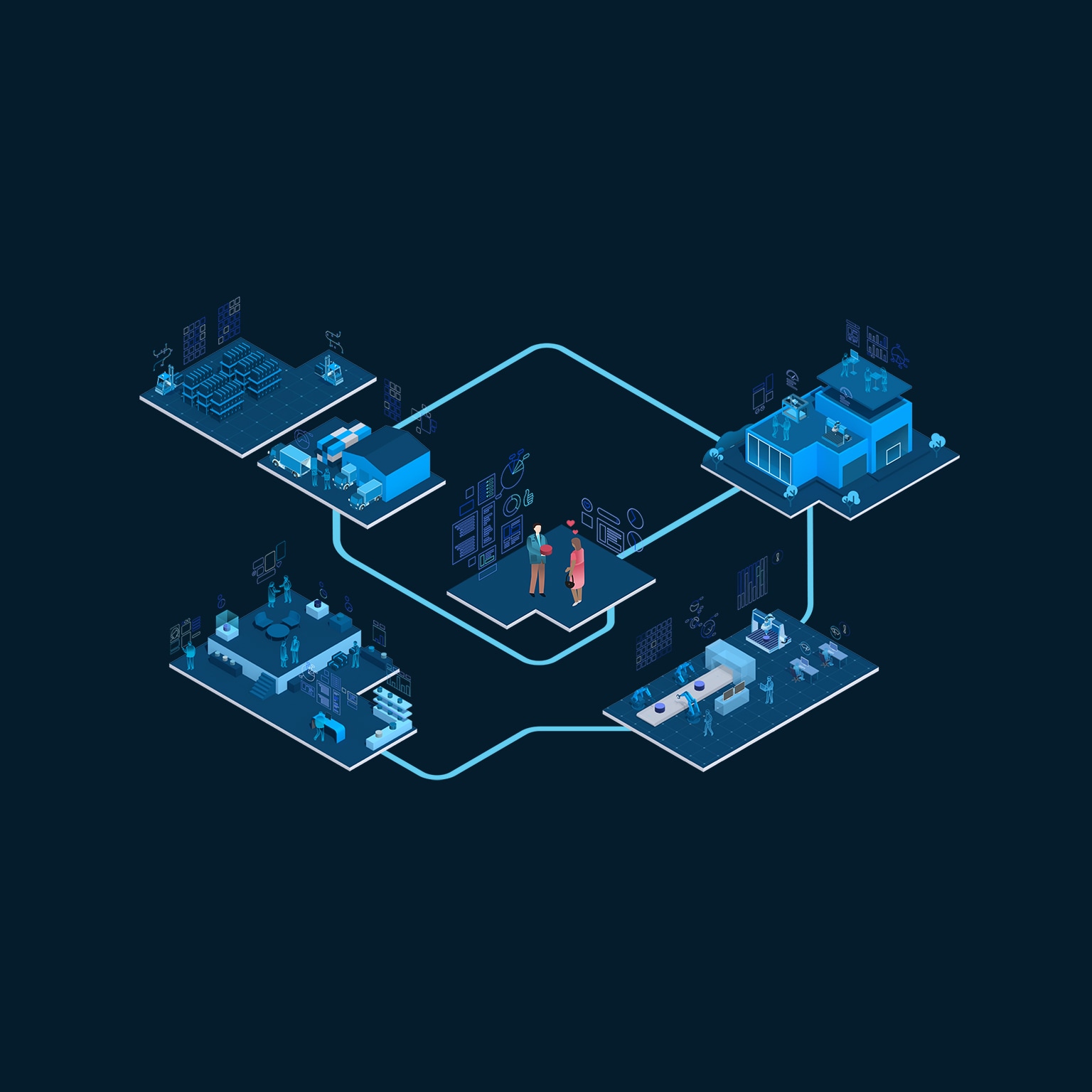

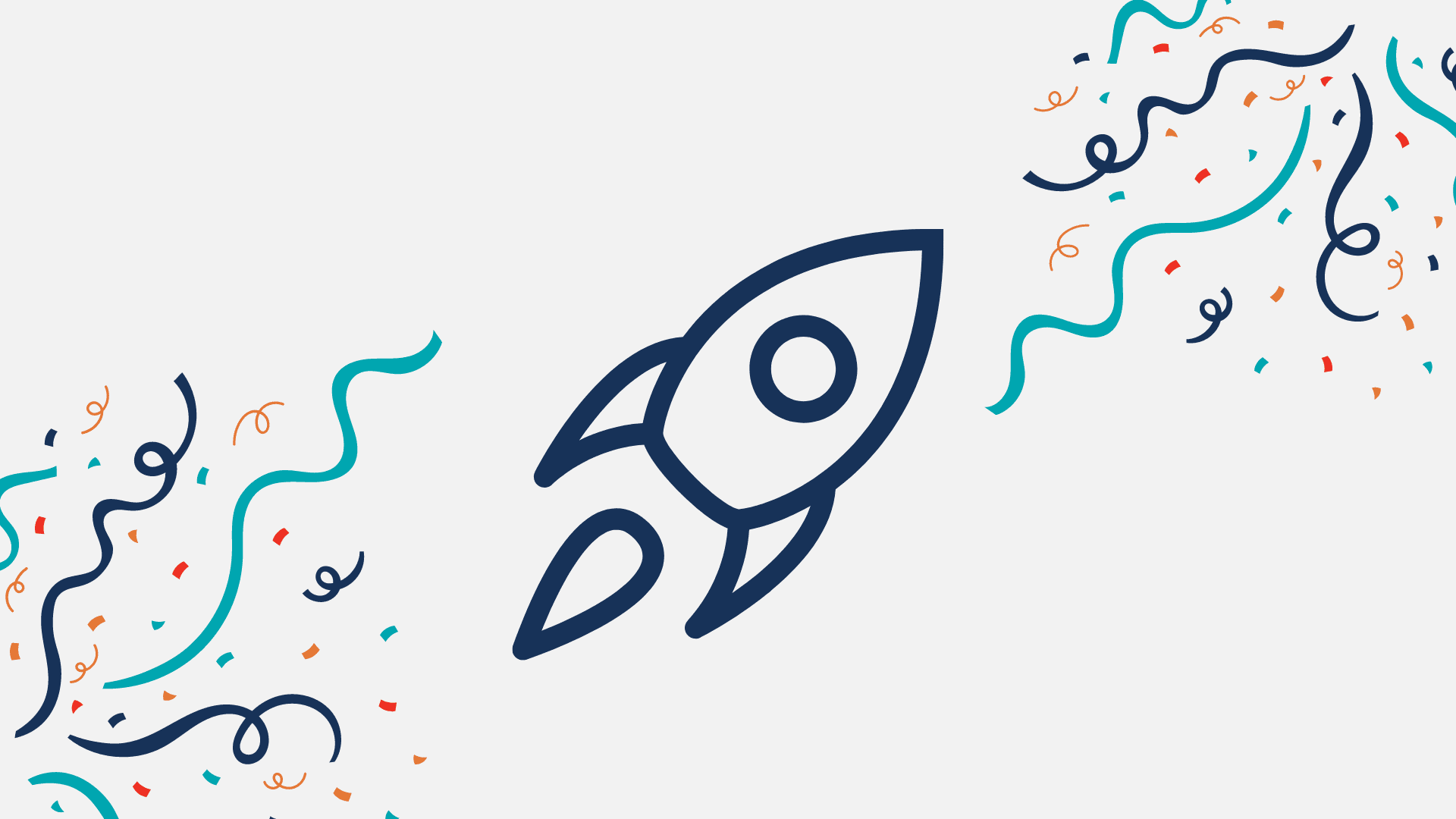
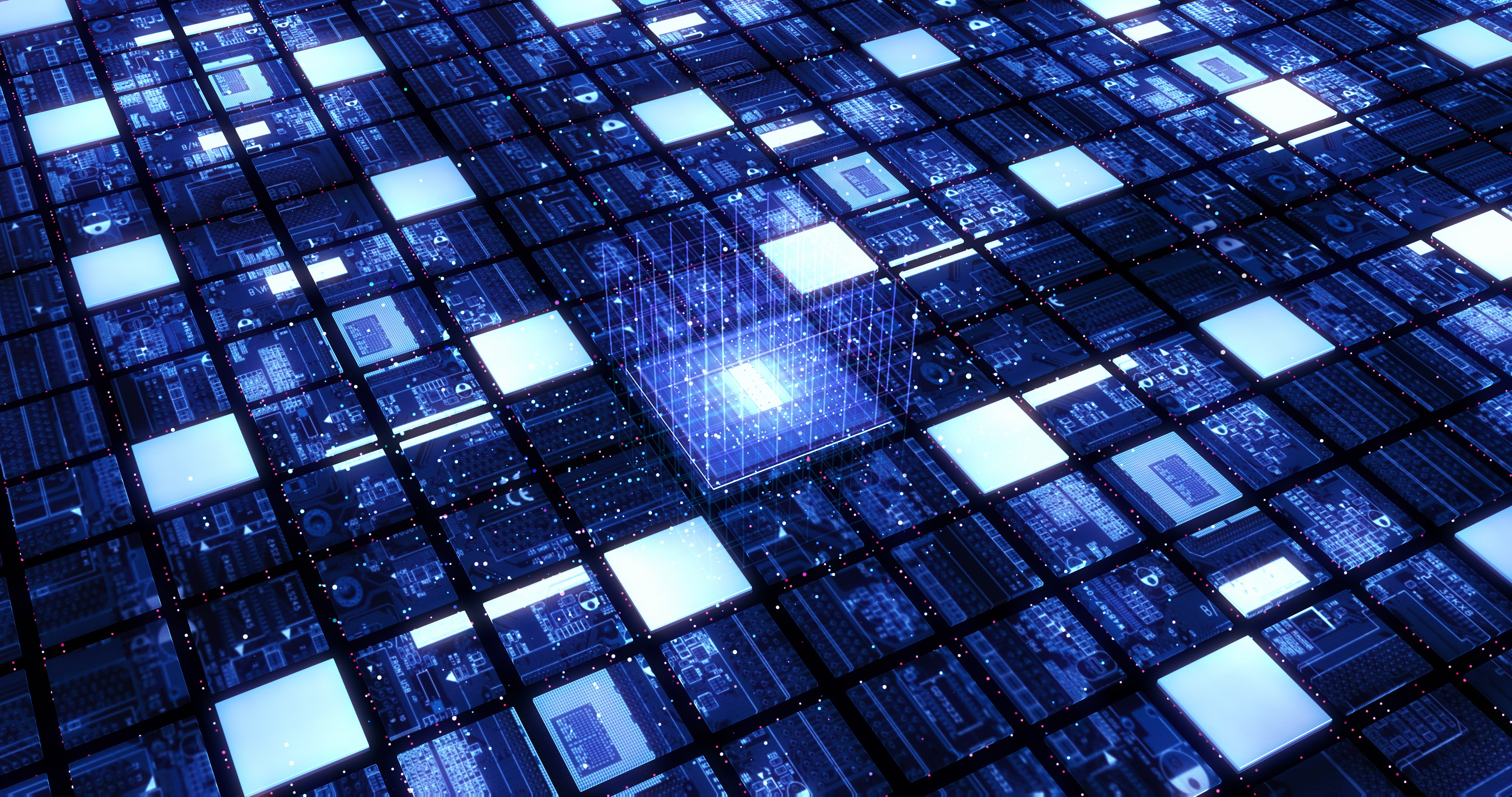
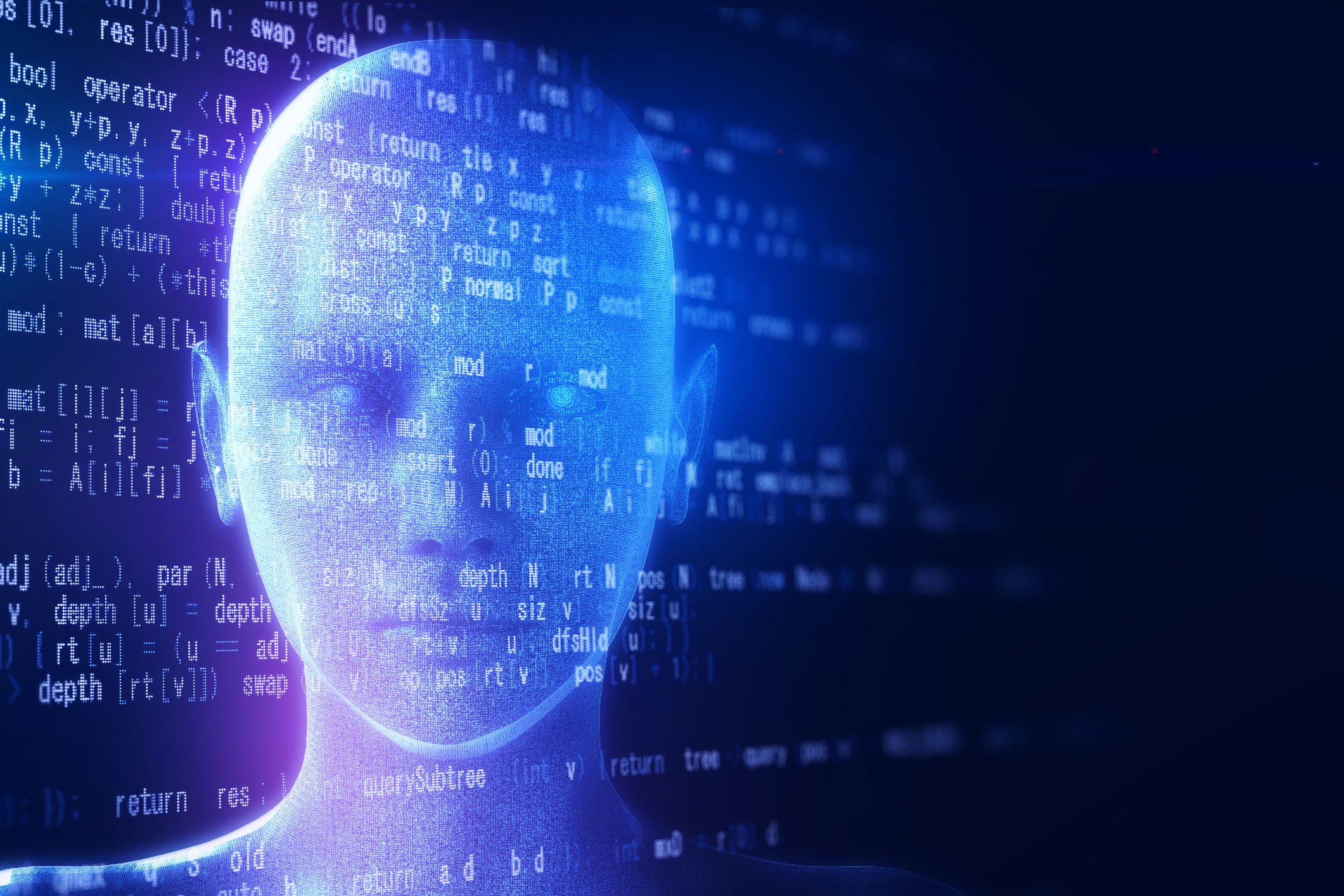